Are you ready to delve into the cutting-edge realm of Generative AI?
Imagine machines that can create art, compose music, or even generate realistic human faces indistinguishable from real ones. Generative AI has revolutionized the way we think about artificial intelligence, pushing boundaries and opening new possibilities.
In this blog, we will take you on a journey to explore everything you need to know about generative AI. From its evolution and types to its top applications, challenges, and future scope, we’ll unravel the fascinating world of this transformative technology.
Get ready to dive into the realm of generative AI and witness the future of creativity and innovation.
Everything You Need to Know about Generative AI
Generative AI has undergone a remarkable evolution since its inception. In the early days of artificial intelligence, the focus was primarily on tasks that required explicit instructions. Machines were limited to performing pre-defined actions based on programmed rules. However, with advancements in machine learning and deep learning techniques, generative AI emerged as a transformative field.
The evolution of generative AI can be traced back to early techniques like Markov Chains and Hidden Markov Models. Markov Chains, based on probability theory, allowed machines to generate sequences of events based on the probabilities of transitioning between states. Hidden Markov Models extended this concept by incorporating unobserved or hidden states, enabling machines to generate sequences with more complex dependencies.
As machine learning techniques advanced, generative AI took a significant leap forward with the introduction of more sophisticated algorithms. Two notable models that revolutionized generative AI are Variational Autoencoders (VAEs) and Generative Adversarial Networks (GANs).
Variational Autoencoders (VAEs) leverage neural networks to encode and decode data. They consist of an encoder that compresses input data into a low-dimensional representation called the latent space and a decoder that reconstructs the original data from the latent space.
VAEs enable machines to learn meaningful representations of data and generate new samples by sampling from the latent space. This approach has been successfully applied in various domains, including image generation, natural language processing, and music composition.
Generative Adversarial Networks (GANs) introduced a novel framework for generative AI. GANs comprise two neural networks: a generator and a discriminator. The generator generates synthetic data samples, while the discriminator tries to distinguish between real and generated data.
Through an adversarial training process, the generator and discriminator compete and improve iteratively. GANs have demonstrated remarkable capabilities in generating realistic images, audio, and text, pushing the boundaries of generative AI.
The evolution of generative AI has paved the way for numerous applications in fields such as art, music, gaming, design, and healthcare. From generating lifelike images and composing original music to creating virtual environments and designing personalized products, generative AI has transformed the way we think about human creativity and innovation.
Types of Generative AI
Generative AI encompasses a diverse range of techniques, each with its unique approach to creative content generation.
Let’s explore some of the prominent types of generative AI and understand their capabilities and applications:
1. Generative Adversarial Networks (GANs)
Generative Adversarial Networks (GANs) have gained significant attention for their ability to generate realistic and diverse outputs. GANs employ a two-network system comprising a generator and a discriminator. The generator network learns to generate synthetic data samples, such as images or text, while the discriminator network tries to distinguish between real and generated data.
Through an adversarial training process, the generator and discriminator compete, resulting in the generation of increasingly authentic outputs. GANs have been successfully applied in image synthesis, video generation, and text generation, among other creative domains.
2. Variational Autoencoders (VAEs)
Variational Autoencoders (VAEs) focus on encoding and decoding data to generate new samples. VAEs consist of an encoder network that learns a compact representation, known as the latent space, from the input data. This latent space captures the essential features and underlying structure of the data.
The decoder network then reconstructs the original data from the latent space, allowing for the generation of novel samples by sampling from the learned latent space. VAEs have found applications in image generation, text generation, and anomaly detection.
3. Autoregressive Models
Autoregressive models generate content sequentially based on previous outputs. These models estimate the conditional probability distribution of the next data point given the previous ones. One popular example of autoregressive models is the Transformer architecture, originally introduced for natural language processing tasks.
Transformers employ self-attention mechanisms to capture contextual dependencies and generate coherent and contextually relevant outputs. Autoregressive models are widely used in natural language generation, music composition, and time series prediction.
4. Reinforcement Learning
Reinforcement Learning (RL) is another approach to generative AI. RL agents learn through trial and error to maximize a reward signal in a given environment. While RL is commonly associated with decision-making and control tasks, it can also be applied to generative tasks.
RL algorithms can learn to generate sequences of actions or outputs that optimize a specific objective, such as game playing, robotic control, or dialogue generation.
Top Applications of Generative AI
Generative AI has become a catalyst for innovation, transforming industries and pushing the boundaries of human creativity.
Let’s explore some of the top applications of generative AI and witness how it is reshaping various domains:
1. Art and Creativity
Generative AI has revolutionized the world of art and creativity. AI-generated artwork, music, and storytelling have gained prominence, with machines collaborating with human artists to produce stunning and unique creations.
AI algorithms can learn from vast collections of artistic works, analyze patterns, styles, and themes, and generate new pieces that exhibit creativity and originality. This fusion of human and machine creativity is opening up new possibilities and challenging traditional notions of art.
2. Gaming Industry
The gaming industry has embraced generative AI to create immersive and realistic experiences. Generative AI algorithms are used to generate virtual worlds, landscapes, and characters, enhancing gameplay and providing players with dynamic and engaging environments.
Procedural generation, a technique employed in generative AI, allows game developers to generate game content, such as levels, maps, and quests, on the fly, ensuring endless variations and exciting gameplay.
3. Healthcare
In the healthcare sector, generative AI plays a vital role in medical image generation and analysis. AI algorithms can generate synthetic medical images that mimic real-world scans, aiding in training and evaluating medical imaging models. Generative AI also assists in drug discovery by generating and optimizing chemical compounds, accelerating the search for potential new drugs.
Additionally, generative AI contributes to personalized medicine by simulating patient-specific physiological responses and optimizing treatment plans.
4. Design and Fashion
Generative AI is revolutionizing the design and fashion industries by automating the creation of unique and personalized products. AI algorithms can generate designs, patterns, and styles based on user preferences, enabling the creation of customized clothing, accessories, and interior designs.
Moreover, generative AI facilitates rapid prototyping and iteration, empowering designers to explore innovative concepts and push the boundaries of creativity.
5. Data Augmentation
Generative AI techniques play a crucial role in data augmentation, especially in the field of machine learning. By generating synthetic data samples that resemble real-world data, generative AI enables the augmentation of training datasets, enhancing the performance and robustness of machine learning models.
Data augmentation techniques help address challenges such as limited labeled data, class imbalance, and generalization.
Challenges in Generative AI
Generative AI offers exciting possibilities for innovation and creativity, but it also poses several challenges that need to be addressed.
Let’s delve into some of the key challenges associated with generative AI:
1. Ethical Use and Misuse of AI-generated Content
One significant challenge in generative AI revolves around the ethical use and potential misuse of AI-generated content. As AI models become capable of creating highly realistic and indistinguishable outputs, concerns arise regarding the ownership and copyright of AI-generated content.
Questions regarding attribution, intellectual property rights, and fair usage need to be carefully addressed to ensure ethical practices and protect the rights of creators and original content producers.
2. Diversity, Fairness, and Interpretability
Generating diverse and representative outputs is crucial to avoid biases and ensure fairness in generative AI. Biases present in the training data can be reflected in the generated content, perpetuating societal or cultural biases. It is essential to develop techniques that encourage diversity and inclusivity in generative AI outputs.
Additionally, ensuring the interpretability of generative AI models is vital to understand the decision-making process and provide explanations for the generated content, particularly in sensitive domains like healthcare or legal applications.
3. Computational Resources and Data Requirements
Generative AI models often demand significant computational resources and vast amounts of training data. Training sophisticated models like GANs or complex neural networks can require extensive computational power, limiting access for individuals or organizations with limited resources.
Acquiring and preprocessing large datasets can also be challenging, particularly in domains where data collection is costly or restricted. Overcoming these barriers requires advancements in hardware capabilities, data availability, and efficient training algorithms.
4. Evaluation and Assessment
Evaluating the quality and assessing the performance of generative AI models is another challenge. Traditional metrics may not capture the nuances of creative outputs or adequately measure the authenticity and uniqueness of the generated content.
Developing reliable evaluation metrics and assessment methodologies specific to generative AI is essential to ensure accurate comparisons, reliable results, and progress in the field.
5. Real-world Adaptation and Robustness
Translating generative AI models from controlled laboratory settings to real-world applications can be challenging. Models trained on specific datasets may struggle to generalize to new and unseen scenarios, resulting in limitations when deployed in practical applications.
Ensuring the robustness, adaptability, and generalization capabilities of generative AI models in real-world environments remains an ongoing challenge.
Future Scope of Generative AI
The future of generative AI holds immense promise and is poised to make a significant impact across multiple industries. As research and development continue to advance, we can expect to witness the emergence of increasingly sophisticated models capable of generating high-quality, diverse, and contextually relevant content.
Let’s explore the exciting future scope of generative AI-
1. Creative Content Generation
Generative AI will continue to push the boundaries of creative content generation. We can anticipate the emergence of AI-generated music hits that captivate audiences worldwide. Machines will collaborate with human artists, writers, and musicians, offering new avenues for creative expression and innovative collaborations.
AI-driven creativity will extend beyond traditional forms of art to encompass new mediums, such as virtual and augmented reality experiences, interactive narratives, and immersive installations.
2. AI-Assisted Creative Collaboration
Generative AI will facilitate enhanced collaboration between humans and machines. AI algorithms will assist artists, designers, and content creators in their creative process, offering intelligent recommendations, generating initial drafts, and providing inspiration based on large-scale analysis of existing works.
This AI-assisted collaboration will foster new levels of innovation, enabling artists and creators to explore uncharted territories and unlock their full creative potential.
3. Personalized Experiences
Generative AI will play a pivotal role in delivering personalized experiences across industries. AI models will analyze vast amounts of user data, preferences, and behaviors to generate tailored content, products, and services. This personalization will be evident in areas such as entertainment, advertising, e-commerce, and healthcare.
Consumers will experience highly customized recommendations, advertisements, and user interfaces, catering to their individual preferences and needs.
4. Healthcare and Medicine
Generative AI will revolutionize the healthcare and medical fields. AI algorithms will aid in medical image generation, enabling realistic and diverse simulations for training and evaluation purposes. AI models will contribute to drug discovery by generating and optimizing chemical compounds, accelerating the development of new therapies and treatments.
Furthermore, generative AI will assist in personalized medicine by simulating patient-specific responses, optimizing treatment plans, and assisting in diagnosis and prognosis.
5. Design and Fashion
Generative AI will continue to disrupt the design and fashion industries. AI algorithms will automate and optimize the creation of unique and personalized products, ranging from clothing and accessories to interior designs and architectural structures.
Customization and personalization will be at the forefront, enabling individuals to have tailored products that reflect their unique style and preferences. AI-driven design tools will empower designers to explore innovative concepts, rapidly iterate designs, and create groundbreaking aesthetics.
6. Natural Language Generation and Conversational AI
Generative AI will advance the capabilities of natural language generation and conversational AI. AI models will be able to generate human-like text, improving chatbots, virtual assistants, and automated customer support.
Conversational agents will exhibit more natural and contextually relevant responses, enhancing user experiences and enabling more effective communication between humans and machines.
In conclusion, generative AI has paved the way for a future where human creativity and AI-driven innovation intertwine. It enables us to explore new realms of creative expression, personalized experiences, and problem-solving.
As generative AI continues to evolve, it is up to researchers, policymakers, and industry leaders to navigate the ethical considerations, address challenges, and unlock its full potential.
By harnessing the power of generative AI responsibly, we can embrace a future where AI augments human creativity and propels us towards new frontiers of imagination and discovery.
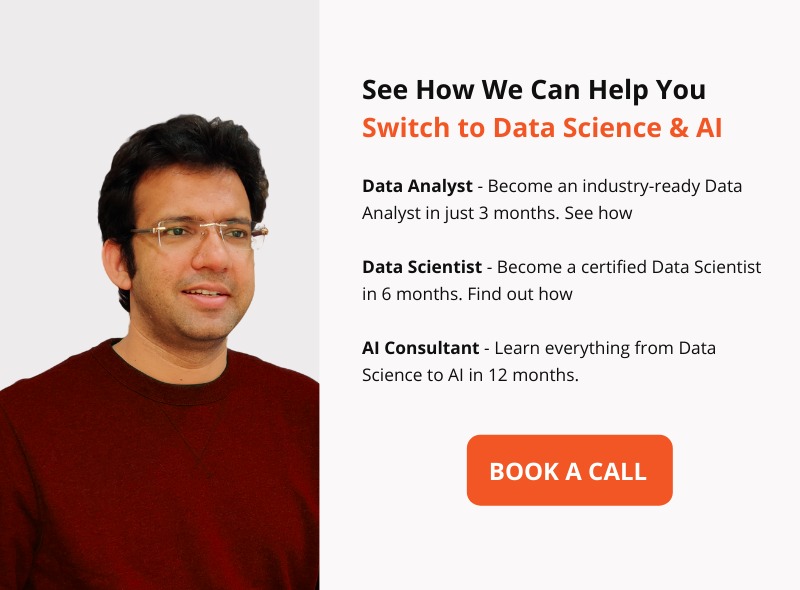
Join ACCREDIAN’s Data Science certifications and start your journey today! With our extensive collection of data resources, pursue a fulfilling career in data science.
Let’s make your data-driven dreams a reality!
Contact us for any questions or comments.