In an era where data reigns supreme, the financial landscape is undergoing a profound transformation. One of the most significant shifts in recent years has been the adoption of credit scoring models in data science to revolutionize the lending industry. These sophisticated models leverage vast amounts of data and cutting-edge algorithms to assess creditworthiness more accurately than ever before.
But, can credit scoring models in data science truly transform lending?
How do these models work their magic to make lending decisions smarter and faster?
What are the implications for borrowers and lenders as this data-driven revolution unfolds?
Join us on this data-driven journey as we delve deep into the world of credit scoring models and uncover how they are reshaping the future of lending.
The Evolution of Credit Scoring Models
Before we dive into the transformative power of data science in lending, let’s take a step back and understand the evolution of credit scoring. Traditional credit scoring relied heavily on factors like credit history, income, and employment status.
While these metrics are valuable, they don’t always provide a holistic view of an individual’s creditworthiness. This is where data science steps in.
Data science has allowed the integration of a myriad of data sources into the credit scoring process. These sources may include social media activity, online behavior, and even data from wearable devices. The result is a much richer and more nuanced understanding of an individual’s financial behavior.
The Role of Machine Learning
At the heart of data-driven credit scoring models lies machine learning. Machine learning algorithms can analyze vast datasets, identifying patterns and relationships that were previously impossible to detect. This ability to recognize subtle correlations allows these models to make more accurate predictions about a borrower’s credit risk.
Some of the key ways in which machine learning is transforming lending include:
- Improved Risk Assessment: Machine learning models can assess risk with unprecedented accuracy, leading to better loan approval decisions.
- Customized Loan Offerings: Lenders can tailor loan products to individual borrowers based on their specific risk profiles, potentially offering lower interest rates to lower-risk applicants.
- Faster Loan Approvals: Automated processes powered by machine learning can drastically reduce the time it takes to approve loans, making it more convenient for borrowers.
- Reduced Bias: Data-driven models can mitigate human biases that sometimes influence traditional lending decisions, resulting in fairer outcomes.
Challenges and Concerns
While the potential of credit scoring models in data science is immense, it’s not without its challenges and concerns.
Some of the key issues include:
- Data Privacy: The collection and use of personal data raises significant privacy concerns. Striking the right balance between data-driven insights and privacy protection is an ongoing challenge.
- Algorithmic Bias: Machine learning models can inherit biases present in the data they are trained on. This raises questions about fairness, especially in lending decisions.
- Interpretability: Some machine learning models are highly complex, making it difficult for borrowers to understand why a particular lending decision was made.
The Future of Lending
The future of lending is undeniably intertwined with data science and credit scoring models.
As technology continues to advance, we can expect:
- Further Automation: Lending processes will become even more automated, reducing paperwork and human intervention.
- Enhanced Customer Experience: Borrowers will benefit from faster approvals, more personalized loan options, and greater transparency.
- Mitigated Risks: Lenders will have better tools to assess and mitigate risks, potentially reducing default rates.
- Regulatory Adaptations: As the industry evolves, regulations will adapt to address data privacy and fairness concerns.
Credit scoring models in data science have the potential to truly transform lending. By leveraging the power of data and machine learning, lenders can make more accurate and efficient lending decisions. However, as with any technological advancement, there are challenges to address, particularly regarding privacy and fairness.
The future of lending will likely see a harmonious blend of human expertise and data-driven insights. Borrowers can look forward to a more streamlined and personalized lending experience, while lenders can better manage risks and make informed decisions.
So, can credit scoring models in data science transform lending? The answer is a resounding yes, and the journey has only just begun. As technology continues to evolve, we can anticipate even more exciting innovations in the lending industry, ultimately benefiting both borrowers and lenders.
Ready to dive deeper into the world of Data Science and AI?
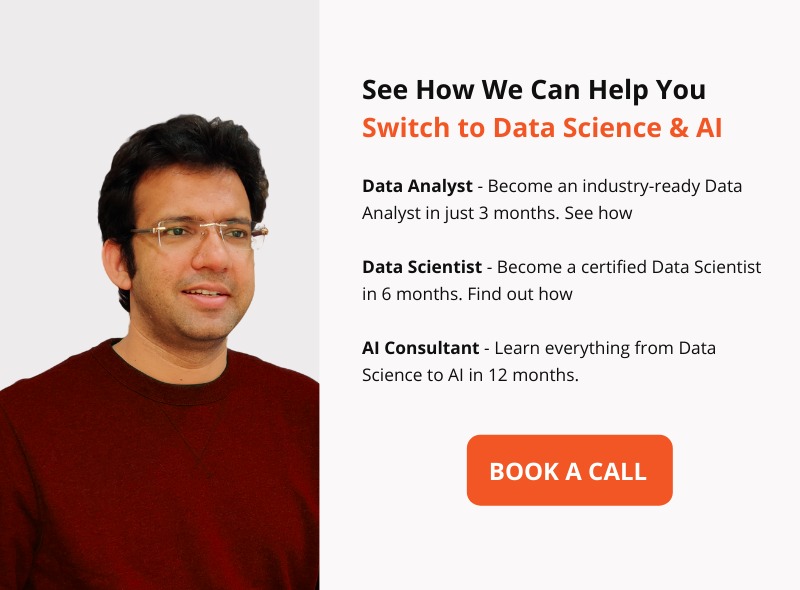
Join Accredian’s Executive Program in Data Science & AI and shape the future of your career. Your transformative learning experience awaits!