Can you imagine accurately predicting future trends, customer behavior, and business outcomes with the power of data?
What if you could make informed decisions that drive growth, optimize operations, and stay ahead of the competition?
Welcome to the world of Predictive Analytics, a game-changing discipline that unlocks the potential of data-driven insights.
In this blog, we will unravel the mysteries of predictive analytics, explore its applications across industries, and equip you with the knowledge to harness its transformative power.
Get ready to dive into the exciting realm of predictive analytics and unleash the hidden potential of your business!
The Ultimate Handbook on Predictive Analytics
In today’s data-driven world, businesses are increasingly turning to predictive analytics to gain valuable insights and make informed decisions about future outcomes.
The core principle behind predictive analytics is the use of advanced statistical modeling techniques to analyze historical data and identify patterns, correlations, and causations. By understanding the relationships within the data, predictive models can make predictions with a certain degree of accuracy.
These models are built based on historical data and trained to recognize patterns that can be generalized to new, unseen data. This process involves selecting appropriate variables, feature engineering, and selecting the most suitable modeling technique for the specific problem at hand.
Predictive analytics has numerous applications across industries. It can be used for sales forecasting to predict future demand, customer behavior analysis to anticipate preferences and buying patterns, risk assessment to identify potential risks and mitigate them, resource optimization to allocate resources efficiently, and much more.
By leveraging historical data and statistical modeling, businesses can gain a competitive edge by making accurate predictions and taking proactive measures to achieve their goals.
The Benefits of Predictive Analytics
Predictive analytics has emerged as a game-changer for businesses across industries, offering a multitude of benefits that can significantly impact their success.
By leveraging the power of predictive analytics, businesses can make informed decisions, optimize resource allocation, improve efficiency, and enhance profitability.
Let’s explore the myriad benefits that predictive analytics brings to the table:
1. Improved Decision-Making
Predictive analytics empowers businesses to make data-driven decisions with a higher degree of confidence. By analyzing historical data and identifying patterns, trends, and correlations, businesses can gain valuable insights into future outcomes. This enables them to make proactive decisions, anticipate market changes, and respond swiftly to emerging opportunities or potential risks.
2. Optimized Resource Allocation
With predictive analytics, businesses can allocate their resources more effectively and efficiently. By accurately forecasting demand, predicting consumer behavior, and understanding market trends, organizations can optimize their inventory management, production planning, and supply chain operations. This helps in avoiding overstocking or understocking situations, reducing costs, and maximizing operational efficiency.
3. Increased Efficiency
Predictive analytics allows businesses to streamline their processes and operations. By identifying inefficiencies, bottlenecks, and areas for improvement, organizations can make data-backed adjustments to their workflows. For example, predictive maintenance can anticipate equipment failures, enabling proactive maintenance schedules and reducing downtime. This leads to improved operational efficiency, minimized disruptions, and enhanced productivity.
4. Enhanced Customer Experience
Understanding customer behavior and preferences is essential for delivering a personalized and engaging customer experience. Predictive analytics enables businesses to analyze customer data and anticipate their needs, preferences, and buying patterns. By identifying cross-selling and upselling opportunities, optimizing marketing campaigns, and providing personalized recommendations, organizations can enhance customer satisfaction, loyalty, and retention.
5. Improved Sales Forecasting
Accurate sales forecasting is crucial for effective business planning and resource management. Predictive analytics enables businesses to project future sales based on historical data, market trends, and other relevant factors. This allows organizations to align their production, inventory, and staffing levels to meet anticipated demand, thereby reducing costs, minimizing inventory holding, and avoiding stock-outs.
6. Risk Mitigation
Predictive analytics helps businesses identify potential risks and take proactive measures to mitigate them. By analyzing historical data and patterns, organizations can assess risks related to fraud, creditworthiness, cybersecurity, and operational disruptions. This enables businesses to implement appropriate risk management strategies, enhance security measures, and make informed decisions to safeguard their operations and assets.
7. Competitive Advantage
In today’s highly competitive landscape, staying ahead of the curve is essential. Predictive analytics provides businesses with a competitive edge by enabling them to anticipate market trends, customer needs, and competitive threats. By leveraging predictive insights, organizations can seize opportunities, differentiate their offerings, and position themselves strategically in the market.
8. Increased Profitability
Ultimately, the overarching benefit of predictive analytics is increased profitability. By optimizing decision-making, resource allocation, operational efficiency, and customer satisfaction, businesses can improve their bottom line. By minimizing costs, identifying revenue-generating opportunities, and reducing risks, organizations can achieve sustainable growth and profitability.
Techniques and Algorithms in Predictive Analytics
Predictive analytics employs a range of techniques and algorithms to analyze historical data and make accurate predictions about future outcomes.
Let’s dive into some commonly used techniques and algorithms, exploring their principles and applications in different scenarios.
1. Regression Analysis
Regression analysis is a statistical tool used to model the relationship between a dependent variable and one or more independent variables. It quantifies the impact of independent variables on the dependent variable and allows for prediction based on observed data.
Linear regression is a popular form of regression analysis, but other variations like polynomial regression or logistic regression can be used depending on the nature of the data and the problem being addressed. Regression analysis is widely applied in sales forecasting, demand prediction, and understanding the relationship between variables.
2. Decision Trees
Decision trees are tree-like structures that represent decisions and their potential consequences. They utilize a hierarchical structure of nodes, where each node represents a decision or a feature to split on.
Decision trees partition the data based on features and make predictions based on the majority class within each partition. Decision trees are interpretable and easy to understand, making them useful for tasks such as customer segmentation, risk assessment, and recommendation systems.
3. Random Forests
Random forests are an ensemble learning method that combines multiple decision trees to make predictions. Each tree in the random forest is trained on a different subset of the data and features, introducing randomness and reducing overfitting. By aggregating the predictions of multiple trees, random forests improve prediction accuracy and robustness. Random forests are widely used in classification tasks, anomaly detection, and feature importance analysis.
4. Neural Networks
Neural networks are a class of algorithms inspired by the structure and function of the human brain. They consist of interconnected nodes, or artificial neurons, organized in layers. Neural networks excel at learning complex patterns and relationships in data through a process called training.
Deep learning, a subset of neural networks, involves training networks with many layers to handle intricate data representations. Neural networks are applied in various fields, including image recognition, natural language processing, and time series forecasting.
5. Support Vector Machines (SVM)
Support Vector Machines (SVM) are supervised learning models used for classification and regression tasks. SVM finds a hyperplane that optimally separates data points into different classes while maximizing the margin between the classes.
SVM can handle both linearly separable and non-linearly separable data through the use of kernel functions. SVMs have applications in sentiment analysis, text classification, and credit scoring.
6. Ensemble Methods
Ensemble methods combine multiple predictive models to make more accurate predictions. Bagging and boosting are popular ensemble techniques. Bagging (Bootstrap Aggregating) trains multiple models on bootstrap samples of the data and combines their predictions.
Boosting, on the other hand, iteratively trains models where each subsequent model focuses on correctly predicting previously misclassified instances. Ensemble methods are effective in tasks such as churn prediction, fraud detection, and recommendation systems.
7. Time Series Analysis
Time series analysis focuses on analyzing and predicting data points that are ordered chronologically. It considers the temporal dependencies and patterns present in the data. Time series analysis encompasses techniques such as autoregressive integrated moving average (ARIMA), exponential smoothing, and state space models. It is extensively used in financial forecasting, stock market analysis, demand forecasting, and weather prediction.
Predictive analytics plays a crucial role in leveraging data for accurate predictions, informed decision-making, and business success. Its potential to drive growth, gain a competitive edge, and unlock the full potential of data cannot be overstated.
Encouraging readers to explore and implement predictive analytics is an invitation to embrace the power of data and leverage it as a strategic asset.
By leveraging predictive analytics, businesses can unlock valuable insights, make informed decisions, and shape a successful future in a data-driven world.
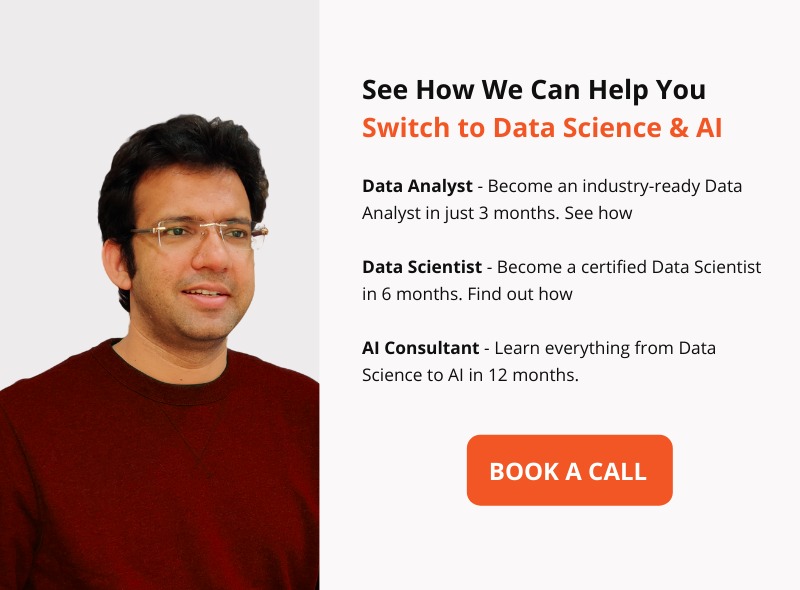
Join ACCREDIAN’s Data Science certifications and start your journey today!
With our extensive collection of data resources, pursue a fulfilling career in data science.
Let’s make your data-driven dreams a reality!
Contact us for any questions or comments.