With the boom in the digital world, Artificial Intelligence (AI) has become an indispensable tool for the FinTech industry. From detecting fraudulent transactions in real time to enhancing risk management strategies, AI is transforming the landscape of financial security.
This evolution is driven by the vast amounts of data generated every second and the need for real-time decision-making in risk assessment and fraud prevention. Here, we explore the ways AI is reshaping risk management and fraud detection, making financial systems smarter, faster, and safer.
Why AI in Risk Management and Fraud Detection?
Financial services are increasingly digital, bringing convenience but also amplifying risk. As the volume of transactions grows, so does the potential for fraud, data breaches, and cyber threats. AI offers a solution by analyzing large datasets, identifying patterns, and predicting potential risks, ensuring that financial institutions remain one step ahead of fraudsters.
Key Benefits of AI in Risk Management and Fraud Detection
- Efficiency and Speed: AI-powered systems can process millions of transactions per second, flagging any unusual activity in real time.
- Improved Accuracy: Machine learning algorithms reduce human error, ensuring accurate detection of fraudulent activities.
- Predictive Analysis: AI can forecast potential risks based on historical data, allowing proactive risk management.
- Cost-Effectiveness: Automated systems reduce the need for extensive manual intervention, cutting down operational costs.
- Enhanced Compliance: AI helps companies comply with regulatory requirements by providing transparency and accountability in risk management practices.
AI in Fraud Detection: How It Works
AI enhances fraud detection by analyzing transaction data, user behavior, and market trends to detect anomalies. Below are some of the primary methods:
-
Machine Learning Algorithms: These algorithms learn from historical data to understand what constitutes “normal” behavior and flag any deviations. For example, if a user’s spending pattern suddenly changes, the system can immediately flag the transaction for review.
-
Behavioral Analysis: By analyzing user behavior patterns, such as login times, location, and transaction habits, AI can determine if an account is potentially compromised.
-
Natural Language Processing (NLP): NLP tools can detect suspicious communications by scanning emails, chats, and other texts to identify potential fraud-related keywords.
These AI tools ensure that any suspicious activity is quickly identified, reducing the time it takes for financial institutions to respond to fraudulent transactions.
AI in Risk Management: Revolutionizing Decision-Making
In risk management, AI plays a crucial role in decision-making by analyzing market trends, economic data, and historical patterns. Here’s how it makes a difference:
-
Predictive Modeling: AI-based predictive models analyze historical data to assess the likelihood of risk. These models consider factors like economic changes, market volatility, and political events that could impact investments.
-
Real-Time Monitoring: AI systems monitor financial markets and detect fluctuations that could signal a potential risk. This allows for quick adjustments in investment strategies and asset management.
-
Credit Scoring: Traditional credit scoring relies on limited information, but AI-powered credit models take into account non-traditional data points, such as social behavior, to provide a more accurate risk assessment.
Real-World Applications of AI in Fraud Detection and Risk Management
-
Credit Card Fraud Detection: AI systems monitor credit card transactions for unusual patterns, such as purchases in different geographic locations within a short time frame.
-
Insurance Fraud: In insurance, AI detects fraudulent claims by analyzing behavioral data and transaction history. For example, if a policyholder files multiple claims in a short time, the system can automatically flag it as suspicious.
-
AML (Anti-Money Laundering) Compliance: AI systems track and analyze transactions to detect money laundering patterns, helping institutions comply with AML regulations.
-
Risk Scoring for Loans: AI improves the accuracy of loan risk assessments by evaluating a wide range of data, including repayment behavior and spending habits, to assign risk scores.
Key Technologies in AI-Driven Fraud Detection and Risk Management
-
Deep Learning: Deep learning, a subset of AI, enables fraud detection systems to improve over time by learning from vast amounts of data, detecting complex patterns, and distinguishing between genuine and fraudulent activities.
-
Big Data Analytics: Big data and AI work in tandem to analyze enormous datasets. AI extracts valuable insights from customer data, improving risk profiling and fraud detection.
-
Robotic Process Automation (RPA): RPA automates repetitive tasks, such as data entry and compliance checks, reducing human error and freeing up time for more complex fraud detection activities.
Challenges of Implementing AI in Financial Security
Despite its benefits, AI in risk management and fraud detection faces several challenges:
-
Data Privacy Concerns: The use of AI requires large datasets, which can lead to privacy issues if not handled carefully.
-
Bias in Algorithms: Machine learning algorithms may develop biases, especially if the training data is unbalanced, leading to inaccurate risk assessments.
-
Regulatory and Compliance Issues: Financial institutions must comply with stringent regulations when using AI, which can slow down innovation.
-
Integration Costs: Implementing AI technology requires significant investment, which may not be feasible for smaller institutions.
The Future of AI in Fraud Detection and Risk Management
The future of AI in fraud detection and risk management looks promising, with new advancements emerging constantly. Here are some expected trends:
-
Enhanced Transparency: AI tools will likely become more transparent and interpretable, allowing regulators and users to understand decision-making processes.
-
Increased Automation: Automation will continue to play a key role, streamlining fraud detection and making real-time responses even faster.
-
More Sophisticated Fraud Techniques: As fraudsters develop more advanced techniques, AI will need to evolve to keep up, making continuous innovation critical.
-
Collaboration with Blockchain: Combining AI and blockchain could offer a more robust system for fraud detection, ensuring data integrity and traceability.
Conclusion
AI in risk management and fraud detection is a game-changer for financial institutions, offering improved efficiency, accuracy, and cost-effectiveness. By utilizing machine learning, predictive analytics, and behavioral analysis, banks and financial services can better protect their customers and their assets.
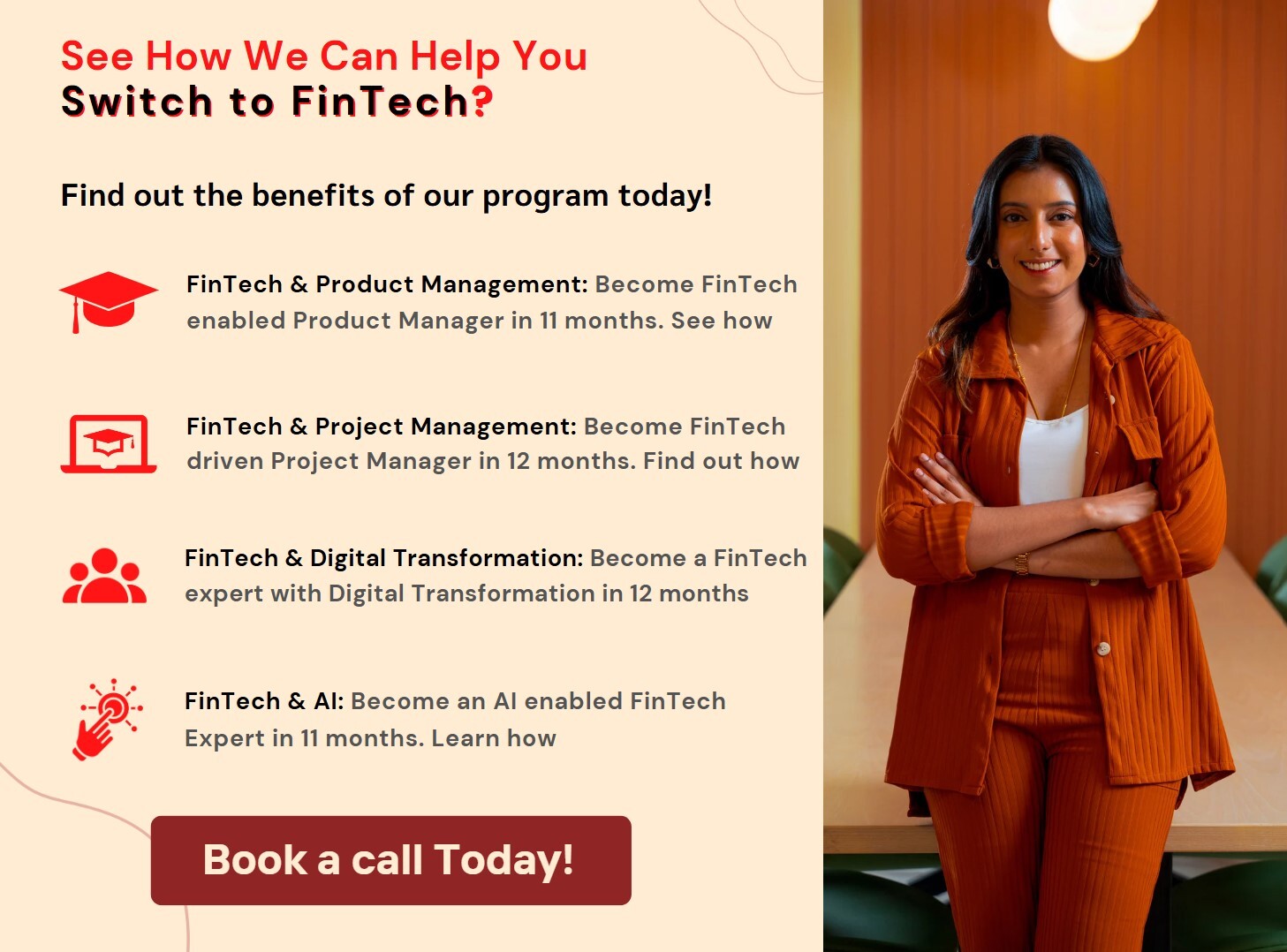
While challenges like data privacy and integration costs remain, the potential benefits far outweigh the drawbacks. As the technology advances, we can expect AI to play an even greater role in securing the financial sector and protecting it from evolving threats.