Ever wondered about the secrets behind the decisions made by AI systems in our daily lives?
As artificial intelligence (AI) continues to shape our world, one concept stands out—Explainable AI (XAI).
How does it unravel the complexity of AI systems?
Join us on a journey through the importance, challenges, and real-world applications of Explainable AI in this comprehensive guide.
Unveiling the Imperative for Explainable AI
The Black Box Conundrum
In the intricate realm of traditional AI models, a persistent challenge emerges—the black box conundrum. These models often operate as enigmatic black boxes, shrouding their decision-making processes in mystery and perplexity. Users find themselves grappling with the elusive nature of how decisions are reached.
Enter Explainable AI (XAI), a beacon of transparency that dispels the opacity surrounding AI decision-making. By unraveling the intricate layers of these black boxes, XAI offers users a profound understanding of the mechanisms driving decisions, fostering trust and clarity.
Ethical Considerations: Navigating the AI Landscape
In a world where AI systems exert influence across pivotal sectors such as finance and healthcare, ethical considerations take center stage. The ethical implications of AI decisions have never been more pronounced or crucial. Here, Explainable AI emerges as a guardian of accountability, wielding the power to address ethical concerns.
By providing users with the tools to comprehend and scrutinize AI decisions, XAI becomes a linchpin in the pursuit of responsible and ethical AI practices. In the realms of finance and healthcare, where the stakes are high, Explainable AI ensures that accountability is not just a buzzword but a tangible reality, empowering users to question and challenge AI decisions when necessary.
The Core Concepts of Explainable AI
1. Model Transparency
At the heart of Explainable AI (XAI) lies the imperative of model transparency. In this realm, the inner workings of AI models are brought into the limelight, shedding the veil of complexity that often surrounds them. The crux of XAI’s effectiveness lies in the development of algorithms and models that transcend mere functionality.
These models not only deliver high performance but also embrace transparency, allowing users to peer into the intricate machinery driving decisions. Through this transparency, XAI ensures that the once-opaque models become comprehensible and, more importantly, trustworthy.
2. Interpretability vs. Explainability
In the nuanced landscape of AI, a crucial distinction emerges—interpretability versus explainability. Interpretability is the art of making a model’s output understandable, providing users with insights into the final outcome. On the other hand, explainability goes a step further, unraveling the intricate web of reasons behind specific decisions.
The key to effective XAI systems lies in striking the delicate balance between interpretability and explainability. By harmonizing these two aspects, XAI ensures not only clarity in the model’s predictions but also a deep understanding of the rationale governing those predictions.
It’s this delicate equilibrium that forms the foundation of XAI’s effectiveness in creating models that are both insightful and comprehensible to users.
Challenges in Implementing Explainable AI
1. Complexity of AI Models
In the ever-evolving landscape of artificial intelligence, the complexity of AI models has surged to new heights. As these models become more intricate, the task of explaining their decisions transforms into a formidable challenge. The crux of this obstacle lies in the intricate web of algorithms and layers that constitute modern AI systems.
Addressing this challenge necessitates the development of innovative methodologies that can distill the complexity of these models without compromising their performance. Striking a delicate balance between simplicity and sophistication becomes paramount, as Explainable AI strives to demystify the enigmatic nature of cutting-edge AI architectures.
2. Trade-off Between Accuracy and Explainability: A Delicate Equilibrium
In the pursuit of optimal AI performance, a perennial challenge surfaces—the trade-off between accuracy and explainability. Often, as models strive for higher accuracy, the veil of complexity thickens, rendering the explanation of decisions more elusive. Striking the right balance becomes an art, requiring thoughtful consideration of the specific use case and the profound impact of AI decisions on end-users.
Explainable AI emerges as the arbiter in this delicate equilibrium, offering a pathway to maintain accuracy without sacrificing transparency. Through strategic considerations and innovative approaches, XAI endeavors to reconcile the inherent tension between accuracy and explainability, ensuring that AI systems are not only high-performing but also comprehensible to those who rely on them.
Real-World Applications of Explainable AI
1. Healthcare
Within the healthcare industry, the integration of Explainable AI (XAI) transcends mere technological innovation—it becomes a beacon of understanding. XAI lends itself to the realm of diagnostics and treatment decisions, offering doctors and patients comprehensible insights into the choices made by AI systems.
This transparency not only cultivates trust but also nurtures a collaborative environment between human experts and AI. In the intricate tapestry of healthcare, XAI emerges as a guiding light, ensuring that the decisions shaping patient care are not just accurate but also understandable to those relying on them.
2. Finance
In the dynamic and consequential world of finance, Explainable AI serves as a powerful tool for shedding light on the decision-making processes that govern investments, risk assessments, and fraud detection. The far-reaching consequences of financial decisions demand accountability and regulatory compliance.
XAI rises to the challenge, providing transparency into the factors influencing these decisions. As a result, financial institutions can navigate the complexities of their operations with a heightened understanding, fostering not only accountability but also a more informed and responsible financial landscape.
3. Autonomous Vehicles
Within the realm of autonomous vehicles, Explainable AI emerges as a critical component in ensuring safety and acceptance. As self-driving cars navigate the roads, XAI unravels the decision-making processes behind their actions. This transparency is paramount for users and regulators alike, as it not only enhances safety but also cultivates trust in the capabilities of autonomous vehicles.
At the intersection of technology and transportation, XAI acts as a bridge, ensuring that the advancements in autonomous vehicles are not just revolutionary but also comprehensible to a world adapting to this transformative technology.
Explainable AI (XAI) transcends the realm of buzzwords, standing as a profound paradigm shift in our approach to and integration of artificial intelligence into the fabric of our lives.
It is not a fleeting trend but rather a fundamental transformation that redefines our relationship with AI technologies.
At its core, XAI addresses a crucial need for transparency in the decision-making processes of AI systems. No longer confined to the mystique of black-box algorithms, XAI unravels the intricacies, making the functioning of AI comprehensible to human users. This transparency is not a mere convenience; it’s a critical shield against the inherent risks associated with the opaqueness of traditional AI models.
By mitigating these risks, Explainable AI lays the foundation for a future characterized by seamless collaboration between humans and machines. In this symbiotic relationship, trust is cultivated as users gain insights into the rationale behind AI decisions.
XAI becomes the bridge that fosters understanding, breaking down barriers that may impede the acceptance and integration of AI technologies into our daily lives.
As we navigate the complex landscape of artificial intelligence, the embrace and understanding of Explainable AI are not mere choices—they are imperatives. In a world where AI systems influence healthcare, finance, transportation, and more, the need for accountability, ethical considerations, and user trust has never been more pronounced. Explainable AI emerges as a necessity, ensuring that the transformative power of AI is harnessed responsibly and inclusively.
In this evolving narrative of human-machine interaction, Explainable AI is not just an option for those at the forefront of technological innovation—it’s the key to unlocking the full potential of AI in a way that aligns with human values and aspirations.
It is a call to embrace a future where the complexities of AI are demystified, and collaboration between humans and machines becomes not just possible but seamless and intuitive.
Ready to dive into the transformative world of Explainable AI?
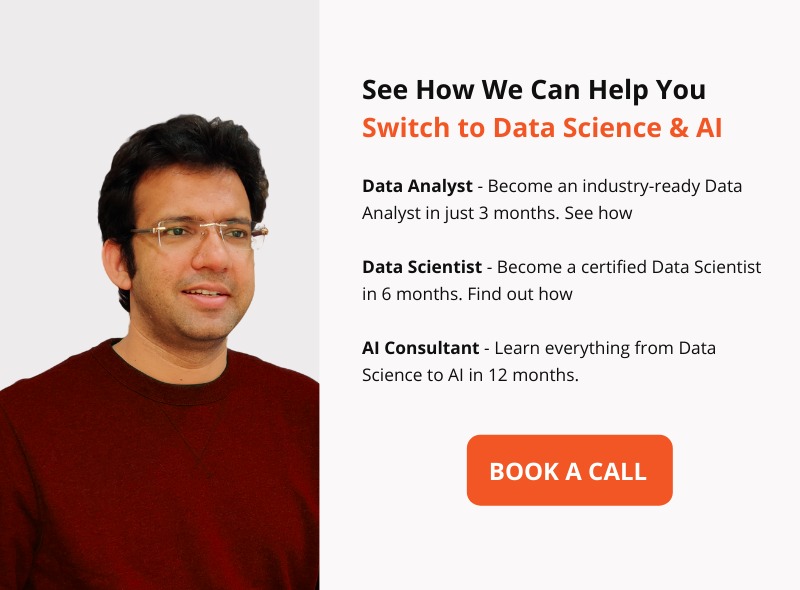
Join Accredian’s Data Science & AI community and be at the forefront of the future. Embrace transparency, mitigate risks, and collaborate seamlessly with our expert-led programs.
Let’s unravel the potential together—join us on the journey to a more comprehensible and responsible AI future!