What if you could communicate effortlessly with your devices, have them understand your emotions, or even create content tailored specifically to your interests?
In recent years, Natural Language Processing (NLP) has evolved at an astonishing pace, transforming the way we interact with technology and data. NLP, a subfield of artificial intelligence (AI), focuses on enabling machines to understand, interpret, and generate human language.
From chatbots and virtual assistants to sentiment analysis and machine translation, NLP is at the heart of numerous applications that enhance our daily lives. This blog will explore the remarkable advancements in NLP and provide a glimpse into the exciting future prospects of this technology.
Basics of NLP
Let’s delve deeper into the fundamental concepts of Natural Language Processing (NLP):
1. Text Classification
Text classification, also known as text categorization, is a fundamental NLP task. It involves assigning predefined categories or labels to a given text based on its content. This process enables machines to automatically organize and sort texts into various groups or topics.
Text classification has a wide range of applications, including:
- Spam Detection: Identifying and filtering out unwanted or spam emails by categorizing them as “spam” or “non-spam.”
- Content Recommendation Systems: Suggesting relevant articles, products, or services to users based on their preferences and past interactions.
- Topic Modeling: Grouping news articles or social media posts into topics like politics, sports, or entertainment for better organization and retrieval.
2. Named Entity Recognition (NER)
NER is another essential NLP task that focuses on locating and classifying named entities within a text. Named entities are specific words or phrases that refer to entities such as names of people, organizations, dates, locations, or monetary values.
NER is crucial for various applications, including:
- Information Retrieval: Enhancing search engines by recognizing and highlighting named entities in search results.
- Knowledge Extraction: Automatically extracting structured information from unstructured text, such as populating a database with facts from news articles or research papers.
- Question Answering Systems: Enabling AI systems to answer questions like, “Who is the CEO of Apple Inc.?” by recognizing the entity “Apple Inc.” as an organization.
3. Sentiment Analysis
Sentiment analysis, also known as opinion mining, is the process of determining the emotional tone or sentiment expressed in a given text. It involves classifying the text as positive, negative, or neutral, and sometimes, more fine-grained sentiments.
Sentiment analysis has various real-world applications, including:
- Customer Feedback Analysis: Analyzing customer reviews, social media comments, and survey responses to gauge customer satisfaction and identify areas for improvement.
- Brand Monitoring: Tracking public sentiment towards a brand or product and identifying PR crises or opportunities for positive engagement.
- Market Research: Understanding consumer sentiment towards a particular product or service, helping businesses make informed decisions.
4. Machine Translation
Machine translation is the task of automatically translating text from one language to another. This is made possible by training NLP models on large multilingual datasets. Machine translation plays a critical role in bridging language barriers and has a variety of applications:
- Global Communication: Facilitating communication between people who speak different languages through tools like Google Translate.
- Content Localization: Adapting content, such as websites or marketing materials, to different languages and cultures for a global audience.
- Cross-Cultural Knowledge Exchange: Enabling the dissemination of information, research, and literature across languages and regions.
Recent Advancements
Let’s explore these recent advancements in Natural Language Processing (NLP) in more detail:
1. Transformer Models
Transformer models, represented by breakthroughs like BERT, GPT-3, and GPT-4, have significantly advanced NLP. These models are built on a novel architecture that employs self-attention mechanisms to process and understand the context of words in a sentence. This allows them to capture long-range dependencies and relationships between words more effectively.
Some key aspects of this advancement include:
- Contextual Understanding: Transformers can grasp the nuanced meaning of a word by considering its context in the entire sentence. This context awareness has led to substantial improvements in various NLP tasks, from text classification to machine translation.
- Transfer Learning: Pretrained transformer models can be fine-tuned for specific tasks, making them versatile and adaptable to a wide range of applications.
- State-of-the-Art Performance: Transformer models have achieved state-of-the-art results in numerous benchmarks and competitions, pushing the boundaries of what NLP can achieve.
2. Zero-shot and Few-shot Learning
One of the remarkable capabilities of transformer models is their ability to perform zero-shot and few-shot learning. Zero-shot learning means the model can handle tasks it has never seen during training, while few-shot learning means it can work effectively with just a small amount of task-specific data.
This is achieved through mechanisms like prompt engineering, where the model is provided with a prompt or a few examples, enabling it to generalize to new tasks.
- Versatility: This advancement makes NLP models highly versatile, as they can adapt to new and unforeseen tasks without retraining, which is especially useful in dynamic and rapidly changing environments.
3. Multilingual NLP
Multilingual NLP models, such as mBERT (multilingual BERT) and XLM-R (Cross-Lingual Language Model for Multilingual Text Classification), have revolutionized cross-lingual NLP tasks. These models can understand and generate text in multiple languages, breaking down language barriers in NLP.
Key aspects include:
- Cross-Lingual Information Retrieval: Multilingual models enable users to search and retrieve information in different languages without language-specific queries.
- Efficiency: Instead of building separate language-specific models, multilingual models are more efficient and cost-effective, as they can handle multiple languages with a single model.
4. Pretrained Word Embeddings
Pretrained word embeddings, such as Word2Vec, FastText, and GloVe, have become integral for NLP models. These embeddings provide a way to represent words in a numerical format, capturing their semantic relationships and contextual information.
Key points include:
- Enhanced Performance: Using pre-trained word embeddings enriches the representation of words, leading to better NLP model performance in tasks like text classification and sentiment analysis.
- Transferability: Pretrained word embeddings can be transferred to different NLP models, making them a valuable resource for various applications.
5. Domain-specific Models
NLP models tailored for specific domains, such as medical, legal, finance, and e-commerce, have become increasingly popular. These domain-specific models are fine-tuned on domain-specific data and can provide more accurate and contextually relevant results.
- Specialization: By focusing on domain-specific language and terminology, these models can outperform general-purpose models in tasks related to their respective domains.
- Industry Applications: Domain-specific NLP models are invaluable in applications like medical diagnosis, legal document analysis, financial sentiment analysis, and personalized e-commerce recommendations.
Future Prospects
Let’s delve deeper into the future prospects of Natural Language Processing (NLP):
1. Explainability and Ethical AI
As NLP models become increasingly powerful, there is a growing need for transparency and ethical considerations in AI development.
Here are some key aspects:
- Model Explainability: Making NLP models more transparent and interpretable is crucial. This helps users understand why a model made a particular decision, especially in critical applications like healthcare or legal analysis.
- Bias Mitigation: Addressing biases in NLP models is a priority. Researchers are working on techniques to reduce and eliminate biases in training data and model outputs to ensure fair and equitable AI.
- Ethical Guidelines: The development of ethical guidelines and standards for AI and NLP is gaining importance. Ensuring that AI technologies adhere to ethical principles is essential to their widespread adoption.
2. Conversational AI
The field of conversational AI is poised for significant growth, with the aim of achieving more human-like interactions.
Key developments include:
- Natural Language Understanding: Improving the ability of chatbots and virtual assistants to comprehend context, emotions, and user intent, making interactions feel more human and productive.
- Emotion Recognition: Integrating emotion recognition into chatbots to provide empathetic responses, particularly in applications like mental health support and customer service.
- Language Generation: Advancements in dialogue generation techniques to enable more coherent and context-aware responses from AI chatbots.
3. Enhanced Multimodal NLP
The integration of text with other modalities such as images and videos offers exciting prospects for NLP.
Some key points include:
- Emotion and Sentiment Analysis: Identifying emotions from a combination of text, images, and videos can have significant applications in mental health support, market research, and content creation.
- Content Summarization: Combining text and visual data to generate concise and informative summaries, which is beneficial for news articles and video content.
- Multimodal Search Engines: Developing search engines that can understand and retrieve information from a variety of modalities, making content discovery more versatile.
4. Real-time Language Translation
With the growth of remote work and global communication, the demand for NLP models that offer real-time, natural-sounding translations is on the rise:
- Live Interpretation: Enabling real-time interpretation during video conferences and meetings, breaking down language barriers in professional settings.
- Travel and Tourism: Facilitating communication for tourists in foreign countries by providing instant translation of spoken and written language.
- Global Collaboration: Supporting seamless collaboration between teams and individuals from different linguistic backgrounds.
5. Personalized Content Generation
The ability of NLP models to generate personalized content for individuals has significant implications for various fields.
Key developments include:
- Customized News Feeds: AI-driven news aggregators that provide personalized news articles tailored to individual interests and preferences.
- Educational Materials: Generating personalized educational content based on a learner’s strengths, weaknesses, and learning style.
- Content Marketing: Creating personalized marketing content to enhance customer engagement and satisfaction.
Natural Language Processing is evolving rapidly, and its impact is felt across various industries. The recent advancements in NLP have set the stage for exciting developments in the future.
With improvements in model performance, explainability, and the integration of multiple modalities, NLP is well on its way to becoming an even more indispensable tool in our everyday lives.
As we look forward, it’s crucial to ensure that ethical considerations are central in NLP development, making AI a force for good that benefits all of humanity.
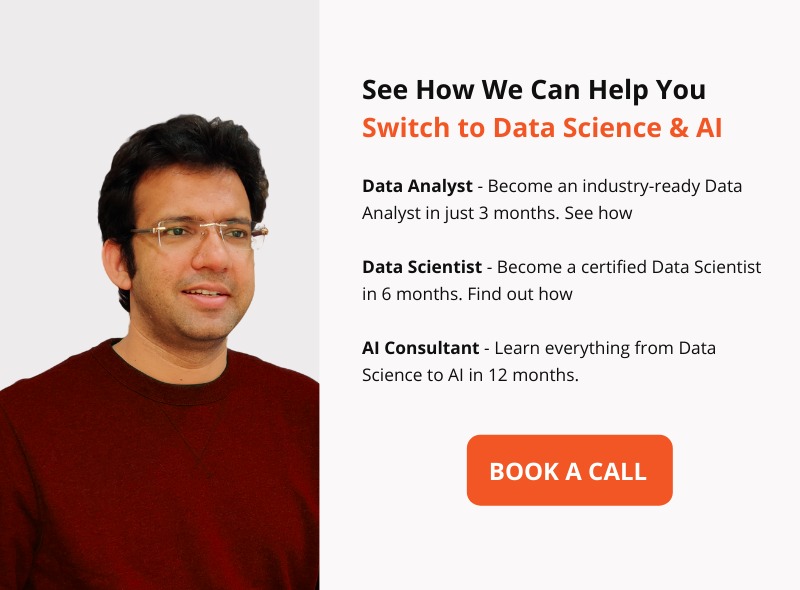
Join Accredian’s Executive Program in Data Science & AI and shape the future of your career. Your transformative learning experience awaits!